Introduction of Generative AI
Generative AI, a subset of artificial intelligence, has emerged as a transformative technology, capable of creating new content and data. From crafting realistic images and composing music to generating human-like text, generative AI reshapes industries and redefines creativity. This article delves into generative AI’s foundational principles and explores its diverse applications across various domains.
Understanding Generative AI

Generative AI models learn patterns from vast amounts of data to generate new, original content. These models are typically built upon deep learning architectures, such as Generative Adversarial Networks (GANs) and Variational Autoencoders (VAEs).
- Generative Adversarial Networks (GANs): GANs consist of two neural networks, a generator, and a discriminator. The generator creates new data instances, while the discriminator evaluates their authenticity. The generator improves its ability to produce realistic output through a competitive process. 1. dasha.ai dasha.ai2. blog.infostrux.com blog.infostrux.com
- Variational Autoencoders (VAEs): VAEs encode input data into a lower-dimensional latent space and then decode it to reconstruct the original data. By sampling from the latent space, VAEs can generate new data instances with similar characteristics.
Foundations of Generative AI
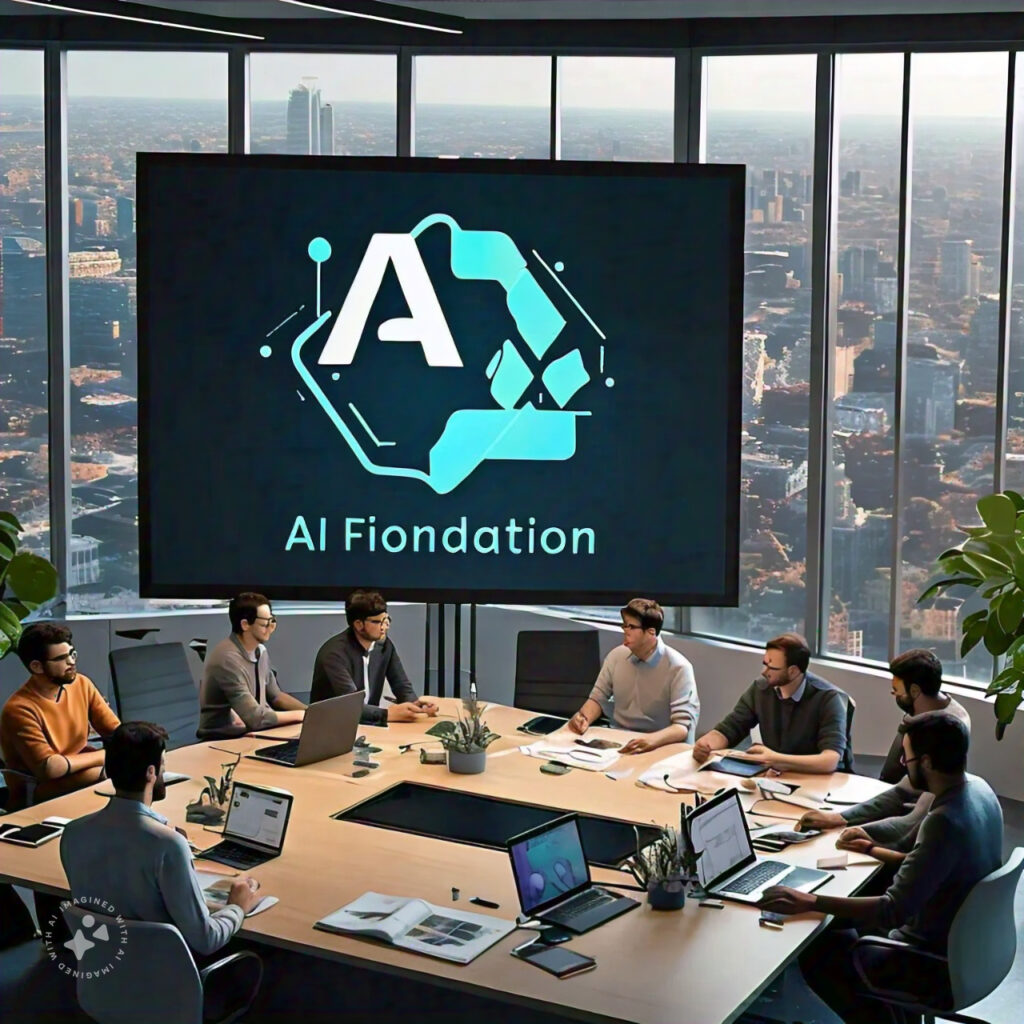
- Data: High-quality and diverse datasets are crucial for training generative AI models. The more data available, the better the model’s ability to learn and generate realistic outputs.
- Deep Learning: Deep learning architectures, particularly convolutional neural networks (CNNs) and recurrent neural networks (RNNs), provide the computational power to process complex patterns in data.
- Algorithms: The choice of algorithm, such as GANs or VAEs, determines the model’s approach to generating new content.
- Computational Resources: Training generative AI models requires significant computational power, often leveraging specialized hardware like GPUs or TPUs.
Applications of Generative AI
Generative AI has found applications in a wide range of fields:
1. Use of Generative AI in Image and Video Generation
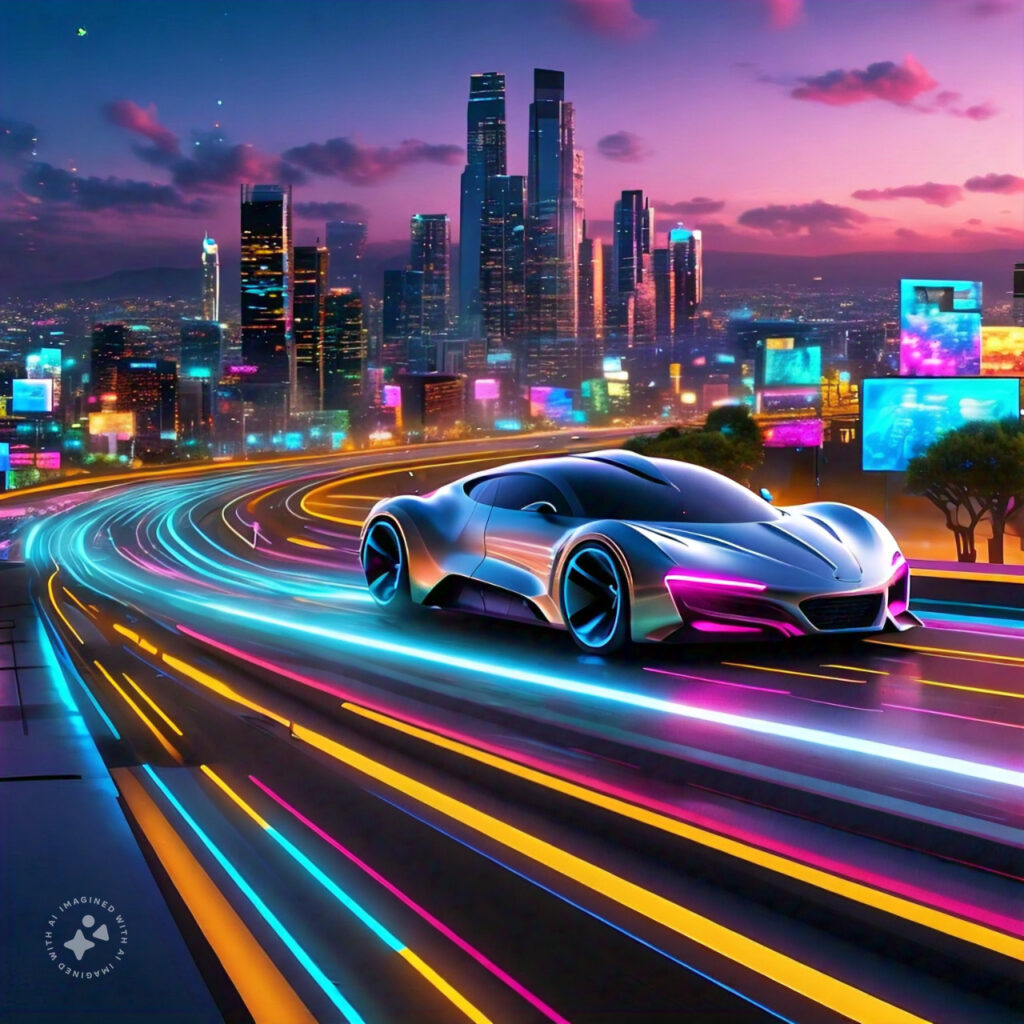
Uses of Generative AI in Image and Video Generation
Image Generation
- Creative Arts: Generating art, fashion designs, and product concepts.
- Advertising: Creating visually appealing ad campaigns.
- Gaming: Generating game assets like characters, environments, and objects.
- Interior Design: Visualizing room designs and furniture arrangements.
- Medical Imaging: Generating synthetic medical images for training AI models.
Video Generation
- Film and Television: Creating special effects, animations, and virtual sets.
- Gaming: Generating in-game cutscenes and cinematic experiences.
- Education: Creating educational videos and simulations.
- Marketing: Generating product demos and explainer videos.
- Virtual Reality: Creating immersive virtual environments.
Common Applications for Both
- Data Augmentation: Generating additional training data for AI models.
- Content Creation: Producing various forms of visual content.
- Simulation: Simulating real-world scenarios for training or testing purposes.
- Entertainment: Creating engaging visual experiences.
2. Use of Generative AI in Natural Language Processing (NLP)
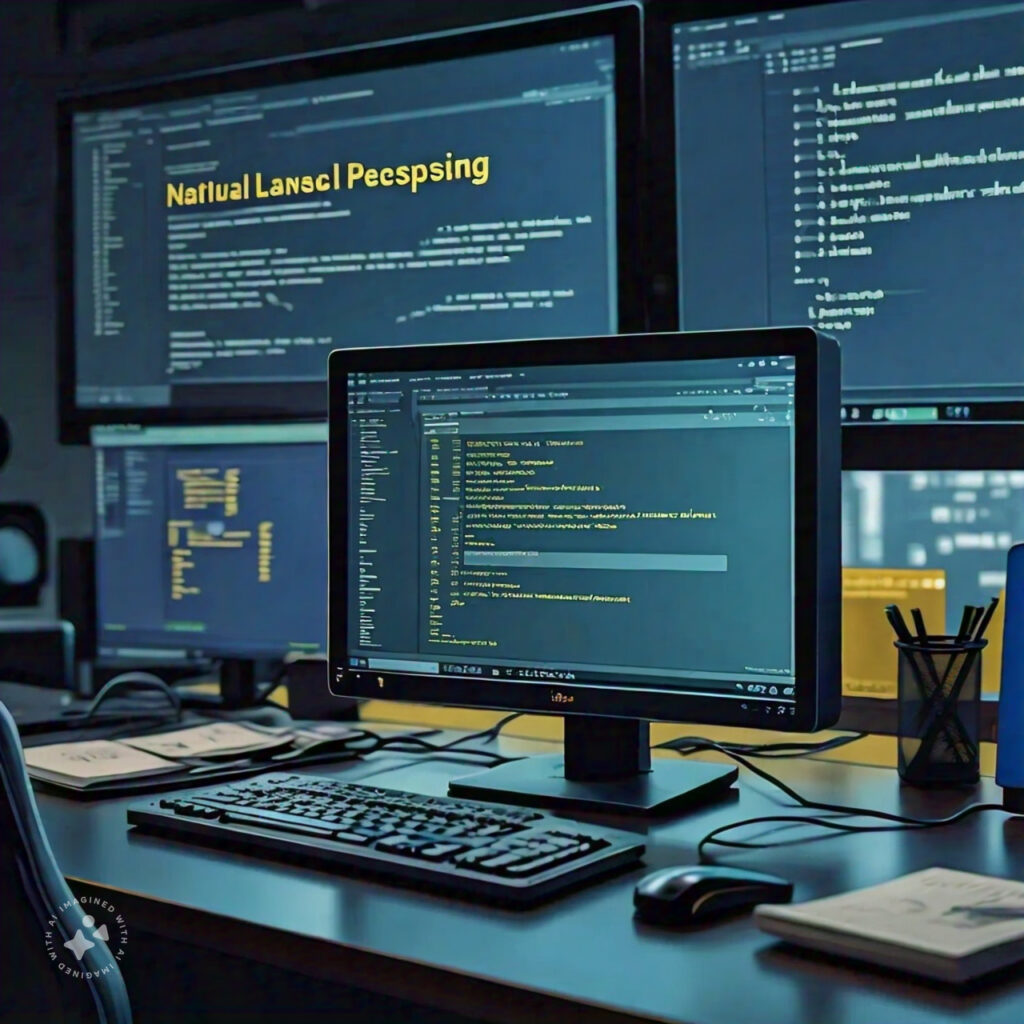
Uses of Generative AI in Natural Language Processing (NLP)
Text Analysis>
- Sentiment analysis: Determining the emotional tone of text (positive, negative, neutral).
- Topic modeling: Identifying the main subjects or themes within a text.
- Text summarization: Condensing long pieces of text into shorter versions.
- Named entity recognition: Identifying and classifying named entities (people, organizations, locations, etc.) within text.
- Keyword extraction: Identifying the most important keywords in a text.
Language Understanding
- Machine translation: Translating text from one language to another.
- Question answering: Providing accurate answers to questions posed in natural language.
- Text generation: Creating new text formats such as summaries, translations, scripts, code, etc.
- Dialogue systems: Enabling computers to communicate and interact with humans using natural language.
- Information retrieval: Finding relevant information from large volumes of text.
Applications of Natural Language Processing
- Chatbots and virtual assistants: Creating conversational agents that can interact with users.
- Search engines: Improving search results by understanding user queries better.
- Social media analysis: Analyzing social media data to understand trends, sentiments, and customer opinions.
- Customer service: Automating customer interactions and improving customer satisfaction.
- Email filtering: Classifying emails as spam or important.
- Language tutoring: Developing language learning applications and tools.
- Medical diagnosis: Processing medical records and reports to assist in diagnosis.
- Legal document analysis: Analyzing legal documents for relevant information and insights.
3. Use of Generative AI in Music and Audio Generation

Music Composition and Generation
- Composition Assistance: AI can help composers by suggesting melodies, harmonies, or chord progressions.
- Style Imitation: AI can mimic the style of specific artists or genres.
- Automatic Composition: AI can generate entire musical pieces without human intervention.
- Personalized Music: AI can create music tailored to individual preferences.
Audio Enhancement
- Noise Reduction: AI can remove unwanted background noise from audio recordings.
- Audio Restoration: AI can restore damaged or degraded audio files.
- Audio Mastering: AI can optimize audio for different platforms and devices.
Sound Design and Effects
- Sound Synthesis: AI can create new and unique sounds.
- Sound Effects Generation: AI can generate realistic sound effects for movies, games, and other media.
- Music Production: AI can assist in creating soundtracks and music production.
Music Analysis
- Music Recommendation: AI can suggest music based on user preferences.
- Music Transcription: AI can convert audio recordings into sheet music.
- Music Emotion Recognition: AI can analyze music to determine its emotional impact.
Voice and Speech
- Text-to-Speech: AI can convert text into natural-sounding speech.
- Voice Cloning: AI can replicate someone’s voice.
- Speech Recognition: AI can accurately transcribe spoken language.
4. Use of Generative AI in Drug Discovery
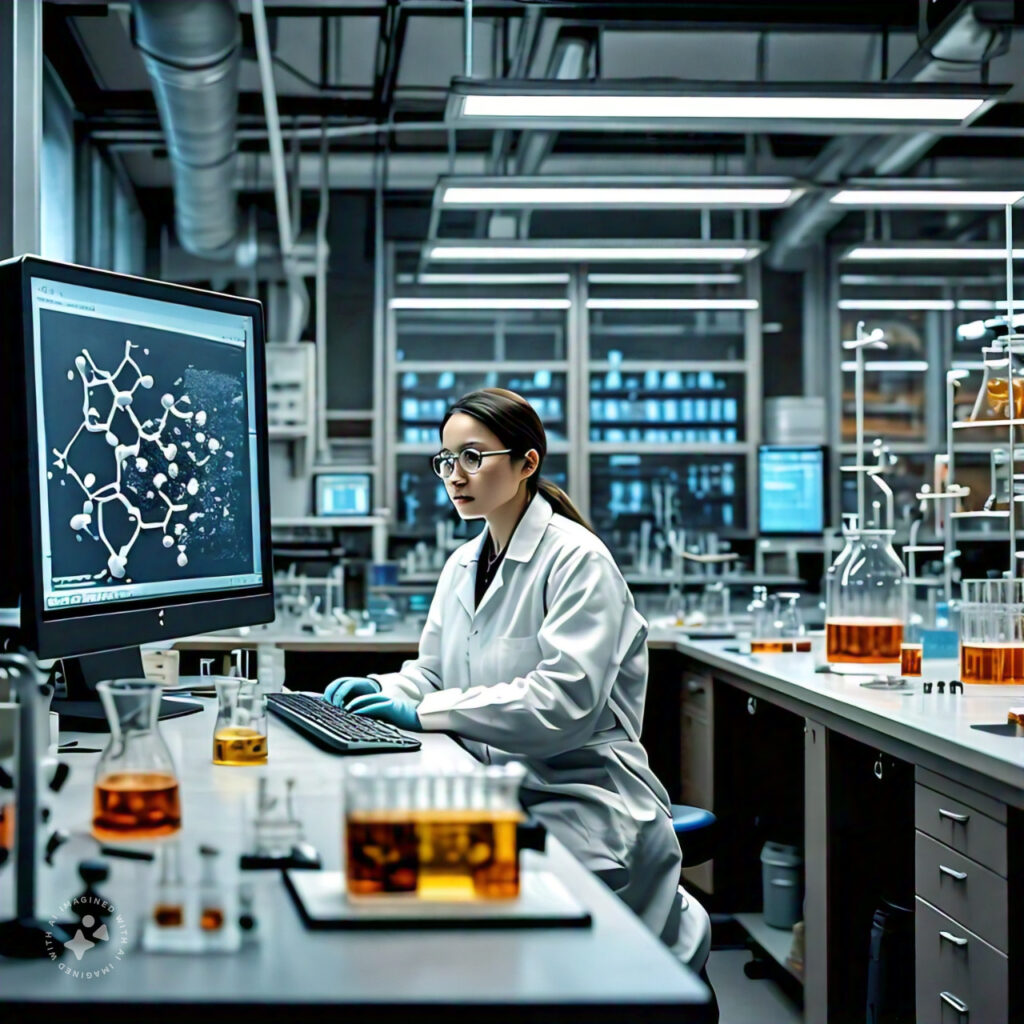
AI is revolutionizing the pharmaceutical industry by accelerating drug discovery and development. Here’s how:
Drug Target Identification
- Identifying disease-relevant proteins: AI can analyze vast biological datasets to pinpoint proteins crucial for disease progression.
- Predicting protein-protein interactions: AI can model how proteins interact, aiding in drug target selection.
Drug Design
- Molecular generation: AI can design new molecules with desired properties.
- Virtual screening: AI can rapidly screen millions of compounds to identify potential drug candidates.
- Structure-based drug design: AI can predict how molecules will interact with target proteins, optimizing drug design.
Drug Development
- Predicting drug properties: AI can estimate drug solubility, bioavailability, toxicity, and other properties.
- Optimizing drug formulations: AI can improve drug delivery and efficacy.
- Clinical trial design: AI can identify optimal patient populations and streamline trial processes.
Drug Repurposing
- Identifying new uses for existing drugs: AI can analyze drug databases to find potential treatments for other diseases.
Other Applications
- Predicting drug interactions: AI can identify potential side effects and drug-drug interactions.
- Accelerating clinical trials: AI can analyze patient data to optimize trial design and recruitment.
- Drug safety monitoring: AI can analyze real-world data to identify potential safety issues.
5. Use of Generative AI in Art and Design

AI is transforming the art and design landscape by offering new tools and possibilities. Here’s a breakdown:
Creative Process Enhancement
- Style Transfer: Applying the style of one image to another, creating unique artistic fusions.
- Image Generation: Creating entirely new images based on given parameters or styles.
- Design Generation: Generating design concepts for products, logos, or interfaces.
- Color Palette Generation: Suggesting harmonious color combinations based on image analysis.
Design Automation
- Image Editing: Automating tasks like removing backgrounds, enhancing images, or retouching.
- Pattern Design: Creating intricate patterns based on given motifs or styles.
- 3D Model Generation: Generating 3D models from 2D images or sketches.
Art Analysis and Authentication
- Art Style Analysis: Identifying artistic styles and influences.
- Art Authentication: Detecting forgeries by analyzing artistic techniques.
- Image Search and Retrieval: Finding similar images or artworks.
Personalized Design
- Custom Design: Creating tailored designs based on individual preferences.
- Product Personalization: Offering customized products based on user data.
New Art Forms
- Generative Art: Creating art through algorithms and data-driven processes.
- Interactive Art: Developing art pieces that respond to user input.
AI is not replacing artists but rather augmenting their capabilities, allowing them to explore new creative horizons and work more efficiently.
6. Role of Generative AI in Gaming Industries

AI has become an indispensable tool in the gaming industry, enhancing gameplay, development efficiency, and player experience. Here’s how:
Enhancing Gameplay
- NPC Behavior: AI powers Non-Player Characters (NPCs) to behave more realistically, making interactions more engaging.
- Game Difficulty: Dynamically adjusts difficulty based on player skill, ensuring a challenging yet enjoyable experience.
- Enemy AI: Creates intelligent and adaptive opponents, providing a sense of challenge and satisfaction.
- Player Behavior Analysis: Understands player preferences to tailor gameplay accordingly.
Game Development
- Level Generation: Automatically generates diverse game levels, saving development time and increasing game replayability.
- Asset Creation: Assists in creating game assets like textures, models, and animations.
- Game Testing: Identifies bugs and imbalances through AI-powered testing.
- Game Balancing: Fine-tunes game mechanics based on player data.
Player Experience
- Personalization: Tailor’s game content and recommendations based on player preferences.
- Chatbots and Virtual Assistants: Provides in-game support and assistance.
- Cheating Prevention: Detects and prevents cheating through AI-powered anti-cheat systems.
- Immersive Experiences: Creates more realistic and immersive game worlds through AI-driven simulations.
Other Applications
- Multiplayer Matching: Matches players with similar skill levels for fair competition.
- Predictive Analytics: Forecasts game trends and player behavior.
- Monetization: Optimizes in-game purchases and advertising based on player data.
AI is continually evolving, and its impact on the gaming industry is expected to grow even stronger in the future.
Challenges and Ethical Considerations
While generative AI offers immense potential, it also presents challenges and ethical concerns:
- Deepfakes: The misuse of generative AI to create realistic but fake media.
- Bias: Generative models can inherit biases present in the training data.
- Copyright infringement: The generation of content that infringes on existing copyrights.
- Job displacement: The potential for automation of tasks traditionally performed by humans.
Addressing these challenges requires a multidisciplinary approach involving researchers, policymakers, and industry leaders.
Conclusion
Generative AI has rapidly evolved from a research concept to a powerful tool with a wide range of applications. As technology continues to advance, we can expect even more groundbreaking innovations in the years to come. By understanding the foundations of generative AI and addressing its challenges, we can harness its potential for the benefit of society while mitigating its risks.